In the ever-evolving landscape of artificial intelligence (AI), one aspect stands out as both a fundamental building block and a transformative force: Search in AI. Imagine a world where machines not only understand human language but can also find information, make decisions, and generate insights like never before. This is the promise of AI search, and in this comprehensive guide, we will delve deep into its intricacies, its real-life applications, and the impact it has on various industries.
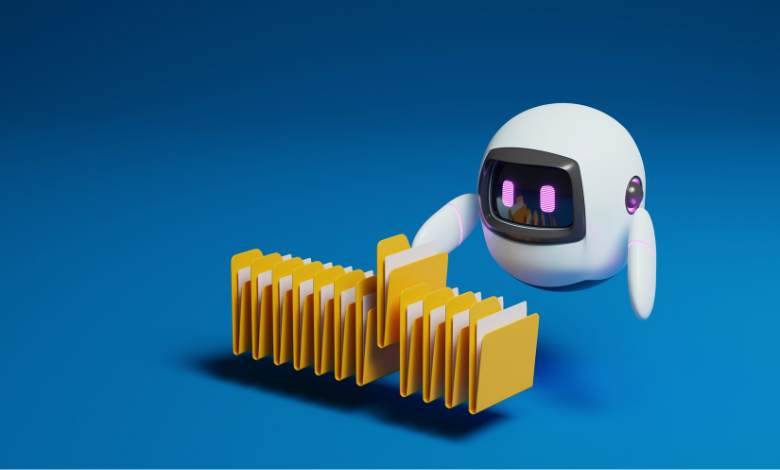
Table of Content
- Understanding AI Search
- Challenges in AI Search
- The Genesis: Keyword Search
- What is Semantic Search?
- How is Semantic Search Different from AI Search?
- Search Engine AI: A Different Beast
- The Role of Generative AI Technologies
- Does AI Search Also Use Large Language Models (LLMs)?
- Navigating the World of Vector Search
- Keywords + Vectors = Hybrid Search
Understanding AI Search
Before we dive into the fascinating world of AI-powered search, let’s grasp the basics. AI search refers to the application of artificial intelligence technologies to enhance the search capabilities of computers and machines. It’s not just about finding relevant documents or web pages; it’s about understanding context, intent, and semantics to deliver meaningful results.
At the heart of AI search is the utilization of advanced techniques like natural language processing (NLP), machine learning, and deep learning. These technologies enable machines to comprehend and interpret human language, making them far more adept at handling complex search queries.
Challenges in AI Search
While AI search holds immense promise, it is not without its challenges. Here are some of the key obstacles that researchers and developers are grappling with:
1. Data Privacy
As AI systems continue to advance in sophistication, their need for access to extensive datasets to enhance performance becomes apparent. This emergence brings forth significant concerns regarding data privacy and security. Navigating the fine line between harnessing the full potential of AI capabilities and safeguarding user privacy is indeed a delicate and challenging endeavor.
2. Bias in Search Results
A search algorithm using artificial intelligence could unintentionally perpetuate bias present in training data. This can lead to biased search results, reinforcing stereotypes and discrimination. Addressing bias in AI search is crucial for fairness and equity.
3. Interpretability
There can be difficulty interpreting AI models that are complex. Understanding why a particular search result was generated or how the AI arrived at a specific decision is crucial for trust and accountability.
The Genesis: Keyword Search
Let’s begin at the very beginning—the traditional keyword search. For years, this has served as the primary approach to discovering information on the internet. You input a word or phrase into a search engine, hit enter, and presto! A list of relevant results appears before your eyes. It’s quick, efficient, and easy to understand. Keyword search relies on matching the exact words or phrases you’ve entered with the content stored on the web. Our search requirements include the digital landscape as well.
What is Semantic Search?
Enter a semantic search. This is where things get intriguing. Semantic search transcends the confines of mere keywords, delving into the profound meanings of words and their contextual search relevance. It aims to understand the intent behind your query and provide results that make more sense in the context of your search. Think of it as a smarter search that doesn’t just consider the words you type but tries to grasp what you truly want to know.
For instance, if you search for “hot dogs,” a keyword-based search might give you a mix of results about sausages, baseball games, and even pet dogs. But a semantic search engine would discern that you’re probably interested in information about the delicious fast food item and prioritize those results.
How is Semantic Search Different from AI Search?
Semantic search and AI search share a strong connection, yet they possess distinct characteristics. While semantic search, as previously explained, revolves around comprehending the essence of your queries, it relies on the application of natural language processing (NLP) techniques to attain this level of comprehension. On the other hand, AI search goes a step further by employing various AI technologies to enhance the search experience.
AI search encompasses a broader range of AI-driven enhancements, including machine learning algorithms, recommendation systems, and personalization features. It adapts and evolves based on user behavior and preferences, making it more intelligent and dynamic than traditional semantic search.
Search Engine AI: A Different Beast
When we talk about search engine AI, we’re talking about the brains behind search giants like Google and Bing. These search engines have integrated AI solutions to provide users with the most relevant results quickly. They employ machine learning algorithms that continuously analyze and improve search results, taking into account factors like user location, search history, and even the device you’re using.
For example, when you search for “best Italian restaurants,” the artificial intelligence (AI) of your search engine may take your location into account and show you nearby options. The power of artificial intelligence is leveraged to improve the search engine experience through personalization.
The Role of Generative AI Technologies
To supercharge AI search, a new player has entered the arena – generative AI technologies. These cutting-edge systems can generate human-like text, making them incredibly useful for understanding and generating content.
Consider the scenario of a user asking a virtual assistant about the latest news. Generative AI can not only understand the query but also generate a summary of recent news articles conversationally and coherently.
This capability is also leveraged in content generation, where AI systems can create blog posts, product descriptions, or even entire news articles. While there’s still a long way to go before they can replicate human creativity entirely, generative AI technologies are making significant strides.
Does AI Search Also Use Large Language Models (LLMs)?
Absolutely! Large Language Models (LLMs) like GPT-3 are at the forefront of AI search. At an unprecedented scale, these models are capable of understanding and generating human-like text. When integrated into search engines or other AI-driven applications, LLMs can provide more accurate and contextually relevant search results.
For instance, if you’re searching for information about climate change, AI search engines powered by LLMs can understand the nuances of your query and provide results that encompass various aspects of the topic, from scientific research to policy discussions.
Navigating the World of Vector Search
Vector search is another fascinating aspect of AI-powered search. Instead of relying solely on keywords or semantic understanding, vector search uses mathematical representations (vectors) of words and documents to find similarities and relationships between them. This approach enables search engines to identify related content more effectively.
For instance, if you’re searching for information on space exploration, a vector search engine might not only consider documents containing the exact phrase “space exploration” but also those that discuss related topics like space agencies, astronauts, and planetary exploration. This multidimensional approach to search leads to more comprehensive and relevant results.
Keywords + Vectors = Hybrid Search
Now, let’s combine the power of keywords and vectors to explore hybrid search. Hybrid search engines take advantage of both traditional keyword-based search and the advanced capabilities of vector search. This marriage of technologies aims to provide users with the best of both worlds – the precision of keyword search and the context-awareness of vector search.
For instance, if you’re looking for a specific recipe, a hybrid search engine can consider the ingredients you have on hand (keyword-based) while also taking into account your dietary preferences and culinary skills (vector-based) to suggest the most suitable recipes.
In summary, “Search in AI” is a multifaceted landscape that encompasses a variety of technologies and approaches, each designed to enhance our ability to find information and make sense of the vast digital world. The possibilities are truly exciting, from semantic search’s focus on understanding intent to generative AI’s content creation abilities, from search engine AI’s personalization to vector search’s contextual awareness, and from LLMs’ language understanding to hybrid search’s combination of strengths.
Our future search experiences will become more intelligent, intuitive, and tailored as we push the limits of AI and search technology. So, the next time you type a query into a search engine, remember that it’s not just a simple keyword match but a sophisticated dance of AI technologies working tirelessly behind the scenes to deliver the information you seek.
In conclusion, “Search in AI” is more than just a buzzword; it’s a revolution in how we interact with information. As we journey deeper into the digital age, AI search solutions like PartsLogic will continue to evolve, making our online experiences more efficient and personalized. Whether you’re a student searching for research material, a traveler looking for the best local cuisine, or a developer seeking code snippets, AI-powered search is here to make your life easier and your results more relevant. Embrace the future of search in AI with innovative services like PartsLogic, and you’ll never look back.