In the expansive digital realm that we navigate daily, search engines have emerged as steadfast companions, guiding us in our quest for answers, unveiling novel insights, and facilitating connections with the global community. Behind the scenes of this seemingly effortless process lies the complex and dynamic concept of “search relevance.” This article dives deep into what search relevance is, why it matters, its historical evolution, and how you can fine-tune it to obtain the most accurate results.
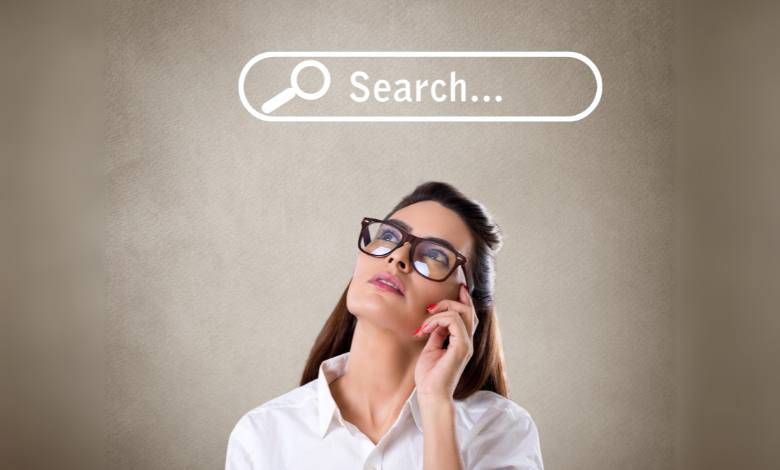
Table of content
- What is Search Relevance?
- Fine-Tuning Search Relevance for Accuracy
- Why Does Search Relevance Matter?
- A Brief History of Search Relevance
- Measuring Relevant Document Search
- The Advancement of Search Algorithms
- Implementing Search Result Boosting and Optimization
- Fine-Tune Your Search Relevance with Partslogic
What is Search Relevance?
A search engine’s success depends on relevance. It is the science and art of presenting users with results that are closely aligned with their queries, ultimately providing the most relevant results for a given search. When you type a question into Google or any other search engine, you expect it to return results that directly address your needs. This precision in delivering what you’re looking for is the essence of search relevance.
Think of it as a librarian who magically knows the exact book you’re seeking in a vast library. Search engines like Google use complex algorithms to understand the intent behind your query, evaluate the content available on the internet, and then present you with a list of websites ranked by their perceived relevance to your question.
Fine-Tuning Search Relevance for Accuracy
Search engines continuously strive to improve the accuracy of their search results. They achieve this by fine-tuning their synonym search relevancy algorithms, which are the sets of rules and criteria used to determine which websites should be displayed and in what order.
To understand the importance of fine-tuning, let’s consider a real-life scenario. Imagine you’re planning a vacation, and you search for the best beaches in the world. The results you receive should ideally include breathtaking beach destinations, travel guides, and recommendations from experts and fellow travelers. However, without a well-tuned search relevance algorithm, you might end up with a list of articles discussing the history of beaches or the geology of sand instead of travel-related information.
Fine-tuning search relevance involves adjusting various factors like keyword matching, user behavior analysis, and the credibility of sources. It’s an ongoing process that aims to keep up with evolving user expectations and the ever-expanding internet.
Why Does Search Relevance Matter?
Search relevance isn’t just a technical term for search engine engineers to ponder. It profoundly affects your online experience and plays a vital role in various aspects of our digital lives.
1. Efficient Information Retrieval
Imagine if search engines weren’t good at delivering relevant results. You’d spend more time sifting through irrelevant information, making your online experience frustrating and inefficient. Accurate search relevance ensures that you quickly find what you need.
2. E-commerce Success
For businesses, particularly those operating online, site search relevance is a game-changer. When potential customers search for products or services, website relevance can determine whether they find your offerings or your competitors’. If your online store doesn’t appear in relevant searches, you miss out on potential sales.
3. User Satisfaction
Search engines are in the business of providing solutions and answers. The more relevant their searches are, the more trust users are likely to place in them and the longer they will stay with them. This, in turn, maintains their dominance in the market.
4. Advertising Effectiveness
Search engines generate a significant portion of their revenue through advertising. If ads are displayed in contexts that are irrelevant or jarring to users, it can negatively impact the effectiveness of advertising campaigns. Ensuring that ads are relevant to users’ searches is crucial for both advertisers and search engines.
A Brief History of Search Relevance
The history of search relevance is a fascinating journey that begins with the early days of the internet and leads us to the sophisticated search engines we rely on today. Here’s a journey into the evolution of search engines and how they deliver the most relevant results.
Search Engines
The concept of search relevance emerged as a response to the rapid growth of content on the internet. In those early days, researchers were grappling with the challenge of finding effective methods for information retrieval. This need for efficient content discovery gave birth to the very first search engines.
Archie and Gopher: Pioneers of Search
In the early 1990s, two significant milestones in the development of modern search relevance systems emerged: Archie and Gopher. McGill University graduate student Archie, who created it in 1990, and University of Minnesota researcher Gopher, who developed it in 1991, were instrumental in expanding information retrieval and search capabilities.
In the early days of the Internet, academics could search the file systems of other institutions via early search engines. However, they were highly technical and required users to possess advanced knowledge of computers and internet concepts.
Web Search Engines
The landscape of search engines began to change as the World Wide Web flourished in the mid-1990s. The introduction of web crawlers, which automatically loaded and updated web pages into search engine indexes, marked a significant shift in how content was searched and accessed.
Excite and Yahoo: Pioneers of Web Search
Web search engines such as Excite (1993) and Yahoo (1994) quickly gained popularity due to their user-friendly interfaces. These platforms introduced some basic statistical models to understand user queries and their relationship to content. However, their relevance ranking was primarily based on the frequency of keywords in web pages.
While these early systems were functional, they had limitations. Their relevance ranking relied solely on keyword frequency and didn’t consider other criteria to assess the quality of web pages. Users were often presented with search results that matched keywords but didn’t necessarily provide the most valuable information.
The Google Revolution
The search engine landscape underwent a seismic shift when Google entered the scene on September 4, 1998, in Menlo Park, California. Google revolutionized search relevance by developing cutting-edge search engine technology that prioritized the most relevant results.
Google’s commitment to delivering relevant search results led to the development of advanced search algorithms. A newer and more powerful machine learning model was incorporated into Google’s search capabilities in the 2000s. These models enabled features like autocomplete search and instant search, making the search experience even more intuitive and user-friendly.
Measuring Relevant Document Search
The need to search within specific websites and databases became increasingly important as the number of websites and online content grew. Traditional ranking systems often relied on algorithms like TF-IDF (Term Frequency-Inverse Document Frequency) to predict document relevance. TF-IDF assessed how often keywords appeared in documents compared to their appearance in the entire repository.
While TF-IDF and similar early approaches were effective for general-purpose document searches, they didn’t fully utilize the additional structure and metadata found on most websites. Modern content includes titles, descriptions, categories, tags, and other keyword-based information that can enhance search relevance.
The Advancement of Search Algorithms
Over time, search engine companies have explored alternative methods to traditional TF-IDF. Some have shifted their focus towards keyword algorithms rather than statistics. Increasing the accuracy and customization of search results is the goal of these innovations.
In the pursuit of better relevance, search engines have adopted sophisticated ranking quality models like nDCG (normalized discounted cumulative gain). By comparing query results ordered according to a specific query, DCG determines how similar they are. Relevance increases with higher scores. Other methods, such as MRR (mean reciprocal rank) and MAP (mean average precision), also contribute to the ongoing quest for improved search relevance.
The Role of Data Quality
Enhancing search relevance isn’t solely about algorithms; the quality of data in the search index plays a crucial role. Keyword and semantic search are only as effective as the quality of the data they analyze. When dealing with missing values or noisy data, data cleansing is essential to ensure search quality. Structured datasets from various sources, such as titles, descriptions, tags, headers, and metadata, significantly impact search quality.
Implementing Search Result Boosting and Optimization
Search engines today prioritize optimizing search relevance for individual sites because of the expanding content and product offerings on websites. Customizing search results to meet specific business needs is essential for success.
Imagine an e-commerce brand with a vast array of product searches and a wide-reaching customer base. To truly excel, it needs to deliver search results that go beyond mere relevance to individual user contexts. This means that search engines must proficiently deal with synonyms, typos, multi-word queries, and even user-generated questions, all while ensuring a seamless and satisfying shopping experience.
In order to understand and make sense of human language, search engines rely on Natural language processing (NLP). As a result, search engines can provide more relevant results and interpret queries more accurately.
Moreover, businesses often have unique requirements, such as promoting seasonal items or high-margin goods. A robust search system should consider these factors and offer a custom ranking that can adapt over time to meet evolving needs.
To improve relevance further, some algorithms take into account attribute importance and keyword proximity. These refinements ensure that search results align more closely with user intent.
Fine-Tune Your Search Relevance with Partslogic
While the strategies mentioned above are essential for optimizing search engine relevance, there’s a new player in the field that promises to take search relevance to the next level: PartsLogic. This innovative tool utilizes advanced machine learning algorithms to analyze and understand the content of websites at an unprecedented level.
PartsLogic goes beyond traditional keyword matching and semantic search. It can identify the most relevant parts of a web page and present them to users directly in search results. For example, if you’re searching for a specific recipe, Partslogic can extract the ingredients and cooking instructions, saving you time and effort.
Furthermore, Partslogic continually adapts and learns from user interactions, ensuring that it provides the most up-to-date and relevant results. As a website owner or content creator, integrating Partslogic into your platform can give you a competitive edge by offering users a more efficient and satisfying search experience.
In conclusion, search relevance is the magic behind the curtain of every search engine, shaping the way we access information and navigate the digital realm. Since keyword matching has evolved into sophisticated algorithms that understand context and intent, it has evolved from being a simple tool to match words. By optimizing their user experience and content quality, businesses and website owners can enhance their online presence. With the emergence of tools like PartsLogic, the future of search relevance promises even more accurate and efficient results, making our online journeys smoother and more satisfying.
As you continue your digital explorations, remember that search relevance is the guiding light that leads you to the internet’s treasures, ensuring that you find what you’re looking for, even in the vast sea of information. So, whether you’re planning a vacation, shopping for the latest gadgets, or simply seeking knowledge, search relevance is your trusted ally in the ever-expanding digital universe.