When you think about search, the first image that likely comes to mind is a person visiting a website and typing a query into a search box. They expect to see search results generated by a search engine that matches their keywords with relevant content in its network architecture. It’s almost second nature to think of search engines as instantly identifying information through keyword matching.
For as long as we can remember, keyword-based search has been the standard. However, the landscape of search technology is undergoing a transformation, and what happens behind the scenes in search applications is evolving. Despite the vast expanse of the internet, keywords are gradually eroding as the primary method of retrieving information. Innovative approaches to search are emerging alongside traditional keyword search, aiming to deliver more precise search results and enhance the overall user experience.
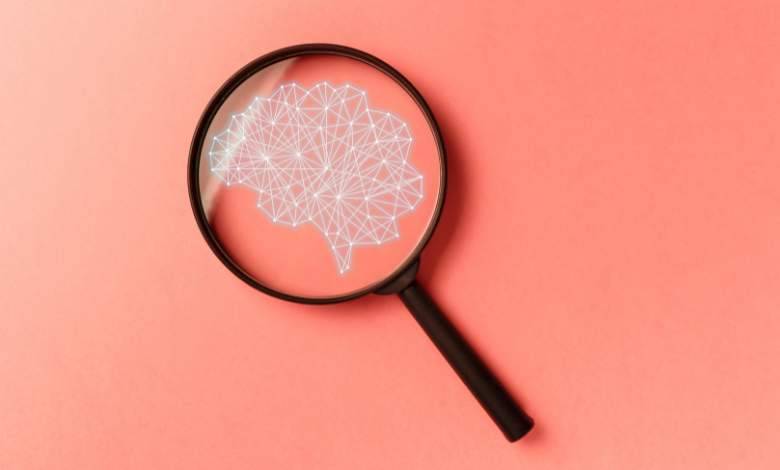
Table of Content
- Traditional Keyword Search
- AI-Powered Search
- Vector Search
- Neural Search
- The Role of Neural Networks and Machine Learning
- How does Neural Search Work?
- The Evolution of Search Engines
- Check out NeuralSearch
Let’s delve into some of the cutting-edge search techniques currently employed by companies worldwide:
Traditional Keyword Search
Before we dive into the intricacies of neural search, let’s first understand the shortcomings of traditional keyword-based search engines. These engines rely on a straightforward method: you input keywords, and they fetch results that contain those exact keywords. While this approach has served us well for decades, it has its downsides.
Conventional search engines frequently pose a challenge to semantic and contextual searches. In other words, they simply match patterns between the words you enter without understanding the meaning behind them. For instance, if you search for “apple,” you might get results about the fruit, the tech giant, or even the record label. These engines also struggle with understanding natural language and nuances, leading to imprecise results.
AI-Powered Search
In the present day, artificial intelligence (AI) is revolutionizing the search engine market. Our interaction with digital information has undergone a radical change as a result of machine learning-powered AI-powered search.
These models, often referred to as Neural Network Search Engines, have the ability to understand the semantics of the query and the content they index, making them remarkably adept at deciphering user intent. They don’t just look for keywords; they strive to understand the meaning behind them.
Vector Search
Numerous modern AI-driven search engines have embraced vector search, harnessing the capabilities of machine-learning models designed to discern semantic relationships.
Search vectors, in this context, serve as mathematical representations of textual data, encompassing search queries and indexed content like product descriptions and web pages. These vectors empower search engines with the ability to fathom the contextual nuances within text. During the process of translating words into vectors, their meanings are encoded.
To illustrate, a conventional keyword-based search engine may not inherently recognize the connections between terms like “warm,” “heat wave,” and “summer.” In stark contrast, vector-based search engines excel at deciphering such intricate word associations, thereby surpassing the limitations of keyword-only searches.
Thanks to this augmented dimension, vectors have become the favored choice for tasks such as automating synonym search identification, document clustering, semantic interpretation of queries, and result ranking.
However, it’s worth noting that, in comparison to output from keyword-centric search engines, vector search may exhibit slower response times, scalability challenges, and higher operational costs.
Neural Search
Enter neural search, the burgeoning star in the realm of search methodologies, poised to revolutionize the search landscape. Though it may sound academic, this approach really involves complex, networked node-based “thinking,” which is carried out by algorithmic elements called artificial neural networks (ANNs) or neural networks. Neural search melds the prowess of vectors, offering precise data representation with expeditious performance and autonomous learning capabilities.
The emergence of Neural Search 101 can be attributed to the era of “Big Data,” without which the abundance of data required for accurate analysis would be lacking. The contemporary data repositories amassed by society enable neural networks to sift through vast troves of information, much of it unstructured, and assimilate it for a multitude of applications.
Enhancing Semantic Comprehension:
Neural search fosters an enhanced semantic grasp of users’ search intentions. It harnesses AI to detect connections between individuals, content, site search data, correlations between user interests, and past and present search queries. It can proficiently learn complex data representations and exploit interrelations between data points, giving it an advantage in comprehending the semantics of search queries.
By proficiently merging semantic and keyword-based retrieval methods, neural search ensures a superior understanding of searchers’ intent, resulting in the provision of more pertinent search results.
Adaptability and Autonomy:
Neural search exhibits adaptability, making it suitable for various data types, including images, videos, audio, and 3D information. For instance, an image’s vector representation can encapsulate details about its shape, color, and content, facilitating effective matching with other images.
Furthermore, neural search streamlines search management, obviating the need for constant oversight. This means website search administrators are relieved of tasks such as adding synonyms, formulating intricate search rules, devising keyword-stuffed titles, or integrating supplementary language resources.
Rather than relying on rule creation and model updates, the neural search commences with a pre-trained neural network, which refines its performance through continuous learning. Its foundation in learning algorithms allows it to adapt to evolving real-world conditions over time via fine-tuning.
In summation, neural search embodies the following attributes:
- Proficiency in understanding search queries.
- Potency.
- Flexibility.
- Suitability for dynamic environments where training data and input continually evolve, such as in the realm of e-commerce.
The Role of Neural Networks and Machine Learning
Neural Search is the neural network, a computational model that draws inspiration from the human brain. Neural networks are comprised of interconnected nodes, akin to artificial neurons, arranged in distinct layers. These networks undergo extensive training on expansive datasets to discern patterns, correlations, and hierarchies within the data.
When it comes to search engines, neural networks play a pivotal role. Machine learning plays a crucial role in enabling search engines to not only grasp keywords but also discern the underlying intent behind a user’s query. Machine learning models, including deep learning, are applied to these neural networks to fine-tune their performance continuously.
Consider a traditional search query like “best smartphones.” A conventional search engine might rely on keyword matching, returning results based on the presence of the exact phrase “best smartphones” on a webpage. Neural search transcends the confines of mere keywords; it discerns that the user’s intent likely revolves around obtaining information concerning highly-rated mobile devices. It can discern this from patterns in data and previous user behavior.
How does Neural Search Work?
Now, let’s dive deeper into the mechanics of neural search. Here’s a simplified step-by-step process:
1. Data Collection and Indexing
Neural search engines start by collecting vast amounts of data from the internet. A website, document, image, or video can be included in this category. The collected data is then indexed, which means it’s organized and stored in a way that allows for quick retrieval.
2. Understanding Queries
When a user enters a search query, the neural search engine doesn’t just look for matching keywords. It analyzes the query’s context, intent, and user preferences. An understanding of the query is obtained using natural language processing (NLP).
3. Neural Network Processing
The neural network takes center stage here. It processes the user’s query and matches it with the indexed data. This matching process considers various factors, including the relevance of content, the credibility of sources, and the recency of information.
4. Ranking and Presentation
After matching the query with the indexed data, the neural search engine ranks the results based on relevance. The user is shown the results that are most relevant to them, frequently along with brief excerpts that give an overview of the material.
5. Continuous Learning
One of the critical advantages of neural search is its ability to learn and adapt. As users interact with the search results—clicking, reading, and ignoring—the neural network learns from this feedback. It has become increasingly adept at delivering personalized and accurate results over time.
The Evolution of Search Engines
The journey from traditional search engines to neural search engines signifies a significant evolution in the digital landscape. As search engines become more sophisticated, users benefit from faster and more accurate results.
Challenges and Future Prospects
Despite the notable strides made by neural search, it encounters its fair share of obstacles. Issues pertaining to privacy, algorithmic bias, and the substantial demand for computational resources represent significant challenges that warrant attention and resolution. Additionally, there is ongoing research into making neural search even more context-aware and personalized.
Check out NeuralSearch
To stay on top of the latest developments in neural search, it’s essential to stay up-to-date with the latest research. If you’re interested in exploring the world of neural search further, be sure to check out Neural Search, a leading platform that harnesses the power of neural networks to deliver cutting-edge search capabilities.
In conclusion, neural search is more than just a buzzword; it’s a technological leap that’s transforming how we interact with the digital world. It’s a testament to the boundless possibilities of AI and machine learning, bringing us closer to the dream of an internet where finding information is not just efficient but deeply satisfying. So, the next time you search for something online, remember that Neural Search is working tirelessly to make your quest for knowledge a seamless and delightful experience.
As the world of search engines continues to evolve, one thing is clear: Neural Search, with its remarkable capabilities, is here to stay and poised for continuous improvement. Embrace the future of search, and let your digital adventures be guided by the power of neural search, powered by PartsLogic.
In the ever-expanding universe of information, neural search stands as our unwavering North Star, illuminating the path to knowledge like never before. With the integration of PartsLogic, it becomes even more potent, offering a refined and efficient search experience. We are not merely searching; we are on a journey of discovery, and with PartsLogic’s enhancement of Neural Search, this voyage looks to be more fruitful and insightful than before.