In our modern digital landscape, inundated with information, businesses encounter the daunting task of captivating and holding the focus of their desired audience. Amid a plethora of choices, consumers frequently grapple with locating offerings that genuinely align with their requirements and inclinations. This is where personalized recommendations step in, revolutionizing the way businesses interact with their customers and enhancing both engagement and conversion rates.
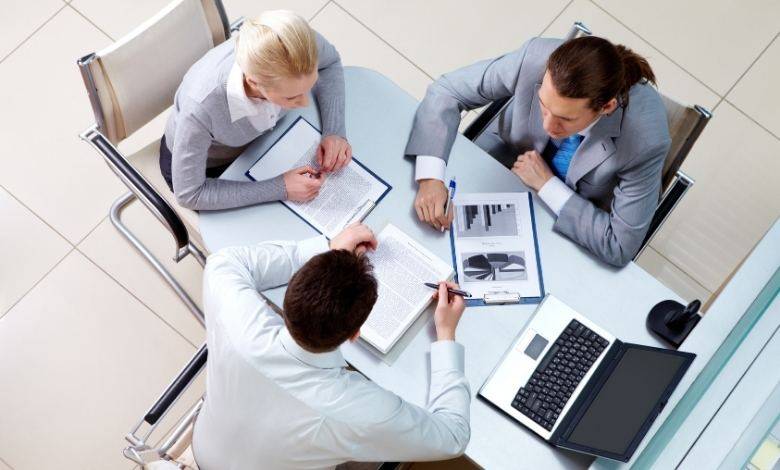
Table of Content
- Understanding Personalized Recommendations
- How Personalized Recommendations Work
- Types of Product Recommendations
- How Can You Use Personalized Recommendations to Lift Engagement, Conversion, and Performance?
- Implementing Personalized Recommendations
- Conclusion
Understanding Personalized Recommendations
Personalized recommendations involve leveraging data about individual users to provide tailored suggestions for products or content that are likely to be of interest to them. This strategy transcends conventional mass marketing methods that simply throw out a wide net, hoping to snag a few interested individuals. Instead, personalized recommendations utilize advanced algorithms and machine learning to analyze user behavior, preferences, and past interactions to deliver relevant and timely suggestions.
Why Personalized Recommendations Matter
In a world where attention spans are shorter than ever and choices are abundant, personalized recommendations emerge as the unsung heroes, guiding us through the maze of options toward products and services that resonate with our individual tastes and preferences. It’s like having a personal shopping assistant who not only understands our needs but also predicts our desires with eerie accuracy.
Personalized recommendations matter because they transform the way individuals engage with products, services, and content online. By leveraging sophisticated algorithms and user data, personalized recommendation systems curate tailored selections that align with each user’s preferences and interests. This customization cuts through the noise of the digital landscape, offering users a more efficient and enjoyable browsing experience. Tailoring suggestions according to previous purchases or viewing history not only boosts user satisfaction but also fosters higher engagement levels. From a business perspective, these recommendations not only increase sales and revenue but also foster deeper connections with customers, leading to greater loyalty and brand affinity. However, it’s essential for businesses to prioritize user privacy and ethical data practices to ensure trust and transparency in their recommendation systems. Overall, personalized recommendations are integral to optimizing the user experience, driving growth, and staying competitive in today’s digital marketplace.
How Personalized Recommendations Work
Personalized recommendation engines employ several techniques to deliver tailored suggestions to users. These techniques include:
Collaborative Filtering
Collaborative filtering stands out as a prevalent method employed in tailoring recommendation systems to individual users. Its operation entails scrutinizing user actions and inclinations to discern correlations and likenesses among users. Based on these insights, the system can recommend products or content that similar users have enjoyed in the past. For instance, should a user buy a specific book, the system might recommend other titles that have appealed to users with comparable purchase histories.
Content-Based Filtering
Content-based filtering prioritizes the intrinsic qualities of products or content over user actions. It involves scrutinizing item attributes like keywords, categories, or descriptions to suggest items akin to those previously favored by the user. For instance, if a user has previously viewed sports shoes, the system may recommend other athletic wear or related accessories.
Hybrid Approaches
Many personalized recommendation systems leverage a blend of collaborative filtering and content-based filtering methods to improve the accuracy of their recommendations. By leveraging the strengths of both approaches, these hybrid systems can provide more nuanced and accurate suggestions that take into account both user preferences and item characteristics.
Types of Product Recommendations
Product recommendation systems employ various techniques to deliver personalized suggestions to users. There are typically three main types of recommendation systems: collaborative filtering, content-based filtering, and hybrid recommendation systems.
Collaborative Filtering Systems:
A collaborative filtering system generates recommendations based on the user’s behavior and preferences. They work by identifying patterns and similarities between users based on their interactions with products or content. By leveraging this collective intelligence, collaborative filtering systems can recommend items that similar users have enjoyed in the past. There are two main approaches to collaborative filtering:
User-based collaborative filtering: User-based collaborative filtering suggests items to users by analyzing the preferences and actions of users with similar profiles. For instance, if User A and User B share comparable purchase histories, items purchased by User B but not by User A might be suggested to User.
Item-based collaborative filtering: Item-based collaborative filtering recommends items to users by examining similarities between items rather than user profiles. For example, if a user expresses interest in a specific item, similar items enjoyed by other users may be recommended.
Content-Based Filtering Systems:
Content-based filtering systems concentrate on the characteristics of items rather than user interactions. These systems analyze the characteristics or features of items, such as product descriptions, tags, or attributes, and recommend items that are similar in content to those that the user has interacted with or shown interest in. Content-based filtering systems prove especially valuable in scenarios where there is scarce or absent user data, as they exclusively depend on item characteristics for recommendations. For example, if a user has viewed or purchased a specific genre of books, a content-based system may recommend other books with similar themes or genres.
Hybrid Recommendation Systems:
Hybrid recommendation systems synergize the merits of collaborative filtering and content-based filtering strategies, amplifying the precision and diversity of suggestions. By blending these approaches, they strive to transcend the constraints of singular methods, offering a comprehensive solution through the fusion of diverse recommendation techniques. The configurations of hybrid recommendation systems can manifest in manifold iterations:
- Weighted hybrid systems: These systems assign different weights to recommendations generated by collaborative filtering and content-based filtering approaches based on their respective strengths and the specific context.
- Switching hybrid systems: These systems dynamically alternate between collaborative filtering and content-based filtering, depending on either user data availability or the nature of the items being recommended.
- Feature combination hybrid systems: These systems merge features from collaborative filtering and content-based filtering methods to create a cohesive recommendation model, encompassing both user preferences and item characteristics.
By leveraging these different types of recommendation systems, businesses can provide more accurate, diverse, and personalized suggestions to users, ultimately enhancing the shopping experience and driving engagement and sales.
How Can You Use Personalized Recommendations to Lift Engagement, Conversion, and Performance?
Personalized recommendations have emerged as a fundamental tactic for businesses spanning diverse sectors, from online retail platforms to streaming services and more. Leveraging personalized recommendations effectively can significantly enhance engagement, conversion rates, and overall performance. Here’s how:
Understanding Customer Preferences: Personalized recommendations rely on data analytics to understand individual customer preferences. By scrutinizing previous transactions, browsing habits, demographic information, and even real-time actions, businesses can acquire valuable insights into the specific interests of individual customers.
Tailored Content Delivery: With a deep understanding of customer preferences, businesses can deliver highly targeted content and product search recommendations. This customized strategy enhances recommendation relevance, boosting customer engagement.
Improved User Experience: Personalized suggestions streamline decision-making, enriching the overall user experience. By presenting customers with options that align with their interests and past behaviors, businesses make it easier for customers to discover products or content they’re likely to enjoy.
Increased Engagement: As customers come across suggestions aligned with their interests, their inclination to interact with the recommended content or products rises. This heightened engagement can result in extended browsing sessions, increased visit frequency, and a stronger bond with the brand.
Higher Conversion Rates: Personalized recommendations can significantly impact conversion rates by guiding customers towards products or content they’re more likely to purchase or interact with. Businesses can gently guide customers toward completing a purchase or engaging in a desired action by strategically offering pertinent suggestions at pivotal moments throughout the customer journey.
Cross-selling and Upselling Opportunities: Personalized recommendations provide opportunities for cross-selling and upselling by suggesting complementary products or premium upgrades based on past purchases or browsing behavior. This not only boosts the average order value but also enriches the overall shopping journey for customers.
Retention and Loyalty: Through the steadfast provision of tailored recommendations that enrich the customer journey, companies can cultivate loyalty and stimulate repeat purchases. Customers who feel acknowledged and attended to are inclined to stay loyal to a brand in the long run.
Continuous Optimization: Personalized recommendation algorithms can be continuously optimized and refined based on feedback and performance data. Businesses can refine their recommendation strategies by analyzing metrics like click-through rates, conversion rates, and engagement metrics, ensuring they provide ever more pertinent and impactful suggestions.
Implementing Personalized Recommendations
While the benefits of personalized recommendations are clear, implementing an effective recommendation system requires careful planning and execution. For businesses interested in leveraging personalized recommendations, here are some key considerations:
Data Collection and Analysis
Personalized recommendation systems must collect and analyze user data to be effective. Businesses must gather relevant data points, such as browsing history, purchase behavior, and demographic information, and use advanced analytics techniques to extract actionable insights. This data-driven approach forms the foundation of personalized recommendations and ensures that suggestions are truly relevant and personalized to each user.
Algorithm Selection and Optimization
For a personalized recommendation system to be successful, the algorithms must be chosen carefully. Businesses must select algorithms that are well-suited to their specific use case and data characteristics, whether it be collaborative filtering, content-based filtering, or a hybrid approach. Additionally, continuous optimization and refinement of these algorithms are necessary to ensure that recommendations remain accurate and effective over time.
User Privacy and Transparency
Businesses must prioritize user privacy and transparency when it comes to personalized recommendation systems. It’s essential to obtain user consent for data collection and clearly communicate how their data will be used to generate recommendations. Empowering users with the ability to manage their data and choose whether or not they receive personalized recommendations cultivates a sense of trust and fosters goodwill among them.
Conclusion
Personalized recommendations represent a powerful tool for businesses looking to enhance engagement and conversion rates in today’s competitive digital landscape. By leveraging advanced algorithms and machine learning methods, personalized recommendation systems can provide customized suggestions that align with users’ preferences and interests. This, in turn, enhances user engagement and boosts conversion rates. As businesses continue to prioritize user-centric experiences, personalized recommendations will play an increasingly vital role in shaping the future of online commerce and content consumption.
And there you have it – a whirlwind tour of the wonderful world of personalized recommendations. From understanding the basics to witnessing real-life examples in action, we’ve covered it all. Next time you’re perusing your go-to online shop or streaming service, pause for a moment to marvel at the wizardry unfolding behind the curtain, all thanks to tailored recommendation algorithms. And if you’re in need of auto parts, don’t forget to give PartsLogic a try. You might just uncover the perfect recommendation that transforms your shopping experience forever.